本篇文章给大家谈谈Pandas/Pyplot中的散点图:如何按类别绘制,以及pyplot散点图的知识点,同时本文还将给你拓展ggplot2PCA散点图绘制、ggplot2~一幅漂亮的散点图和新学到的配
本篇文章给大家谈谈Pandas/Pyplot中的散点图:如何按类别绘制,以及pyplot 散点图的知识点,同时本文还将给你拓展ggplot2 PCA散点图绘制、ggplot2~一幅漂亮的散点图和新学到的配色方案、ggplot,牛图;带有边际直方图的散点图:轴不匹配、Matplotlib 可视化50图:散点图(1)等相关知识,希望对各位有所帮助,不要忘了收藏本站喔。
本文目录一览:- Pandas/Pyplot中的散点图:如何按类别绘制(pyplot 散点图)
- ggplot2 PCA散点图绘制
- ggplot2~一幅漂亮的散点图和新学到的配色方案
- ggplot,牛图;带有边际直方图的散点图:轴不匹配
- Matplotlib 可视化50图:散点图(1)
Pandas/Pyplot中的散点图:如何按类别绘制(pyplot 散点图)
我正在尝试使用Pandas DataFrame对象在pyplot中制作一个简单的散点图,但是想要一种有效的方式来绘制两个变量,但要用第三列(键)来指定符号。我已经尝试过使用df.groupby的各种方法,但是没有成功。下面是一个示例df脚本。这会根据“ key1”为标记着色,但是我想看到带有“ key1”类别的图例。我靠近吗?谢谢。
import numpy as npimport pandas as pdimport matplotlib.pyplot as pltdf = pd.DataFrame(np.random.normal(10,1,30).reshape(10,3), index = pd.date_range(''2010-01-01'', freq = ''M'', periods = 10), columns = (''one'', ''two'', ''three''))df[''key1''] = (4,4,4,6,6,6,8,8,8,8)fig1 = plt.figure(1)ax1 = fig1.add_subplot(111)ax1.scatter(df[''one''], df[''two''], marker = ''o'', c = df[''key1''], alpha = 0.8)plt.show()
答案1
小编典典你可以使用scatter
它,但是这需要为你提供数值key1
,并且你不会注意到图例。
最好只plot
对像这样的离散类别使用。例如:
import matplotlib.pyplot as pltimport numpy as npimport pandas as pdnp.random.seed(1974)# Generate Datanum = 20x, y = np.random.random((2, num))labels = np.random.choice([''a'', ''b'', ''c''], num)df = pd.DataFrame(dict(x=x, y=y, label=labels))groups = df.groupby(''label'')# Plotfig, ax = plt.subplots()ax.margins(0.05) # Optional, just adds 5% padding to the autoscalingfor name, group in groups: ax.plot(group.x, group.y, marker=''o'', line'', ms=12, label=name)ax.legend()plt.show()
如果你希望外观看起来像默认pandas样式,则只需rcParams使用pandas样式表进行更新,并使用其颜色生成器即可。(我也略微调整了图例):
import matplotlib.pyplot as pltimport numpy as npimport pandas as pdnp.random.seed(1974)# Generate Datanum = 20x, y = np.random.random((2, num))labels = np.random.choice([''a'', ''b'', ''c''], num)df = pd.DataFrame(dict(x=x, y=y, label=labels))groups = df.groupby(''label'')# Plotplt.rcParams.update(pd.tools.plotting.mpl_stylesheet)colors = pd.tools.plotting._get_standard_colors(len(groups), color_type=''random'')fig, ax = plt.subplots()ax.set_color_cycle(colors)ax.margins(0.05)for name, group in groups: ax.plot(group.x, group.y, marker=''o'', line'', ms=12, label=name)ax.legend(numpoints=1, loc=''upper left'')plt.show()
ggplot2 PCA散点图绘制
分别利用颜色(colour)和形状(shape i.e. pch)进行分组很多属性需要单独设置。
用到的对象有
- 数据映射(Aes,Data aesthetic mappings)
- 几何属性(Geom,Geometric objects)
- 统计转换(Stat,Statistical transformations)
- 标度(Scales)
- 坐标系(xlab, ylab, coor, coordinate system)
- 主题(theme)
暂时未用到分面(faceting)
myData <- read.csv("data.csv")
myData2 <- myData[,-20]
myData3 <- na.omit(myData2)
pr<-prcomp(myData3[,5:20],scale=TRUE)
pca <- pr$x
####一下为ggplot2绘图
library(ggplot2)
pca <- as.data.frame(pca)
pca$salinity <- myData3$Salinity
pca$climate <- myData3$Climate
pca$ecotype <- myData3$Ecotype
pca$name <- myData3$Plant.name
theme<-theme(panel.background = element_blank(),
panel.border=element_rect(fill=NA),
panel.grid.major = element_blank(),
panel.grid.minor = element_blank(),
strip.background=element_blank(),
axis.text.x=element_text(colour="black"),
axis.text.y=element_text(colour="black"),
axis.ticks=element_line(colour="black"),
plot.margin=unit(c(1,1,1,1),"line"),
legend.title=element_blank(),
legend.key = element_rect(fill = "white")
)
percentage <- round(pr$sdev / sum(pr$sdev) * 100, 2)
percentage <- paste( colnames(pca), "(", paste( as.character(percentage), "%", ")", sep="") )
p <- ggplot(pca, aes(x=PC1, y=PC2,shape=paste(salinity, climate), colour=ecotype,label=name))
#p <- p + geom_point()+geom_text(size=3) + theme
p <- p + geom_point(size=3) +
theme +
xlab(percentage[1]) +
ylab(percentage[2]) +
scale_shape_manual(values = c(15,17,0,2),labels=c("Salt in Fanggan","Salt in Panjin","Fresh in Fanggan","Fresh in Panjin"))+
stat_ellipse(aes(shape = NULL))+
scale_x_continuous(limits = c(-6,6))+
scale_y_continuous(limits = c(-5,5.5))
p
ggplot2~一幅漂亮的散点图和新学到的配色方案
今天在找R shiny的教程的时候发现了一幅比较漂亮的散点图,配色很好看,代码记录在这里。
先贴出结果图,就是这样式的!
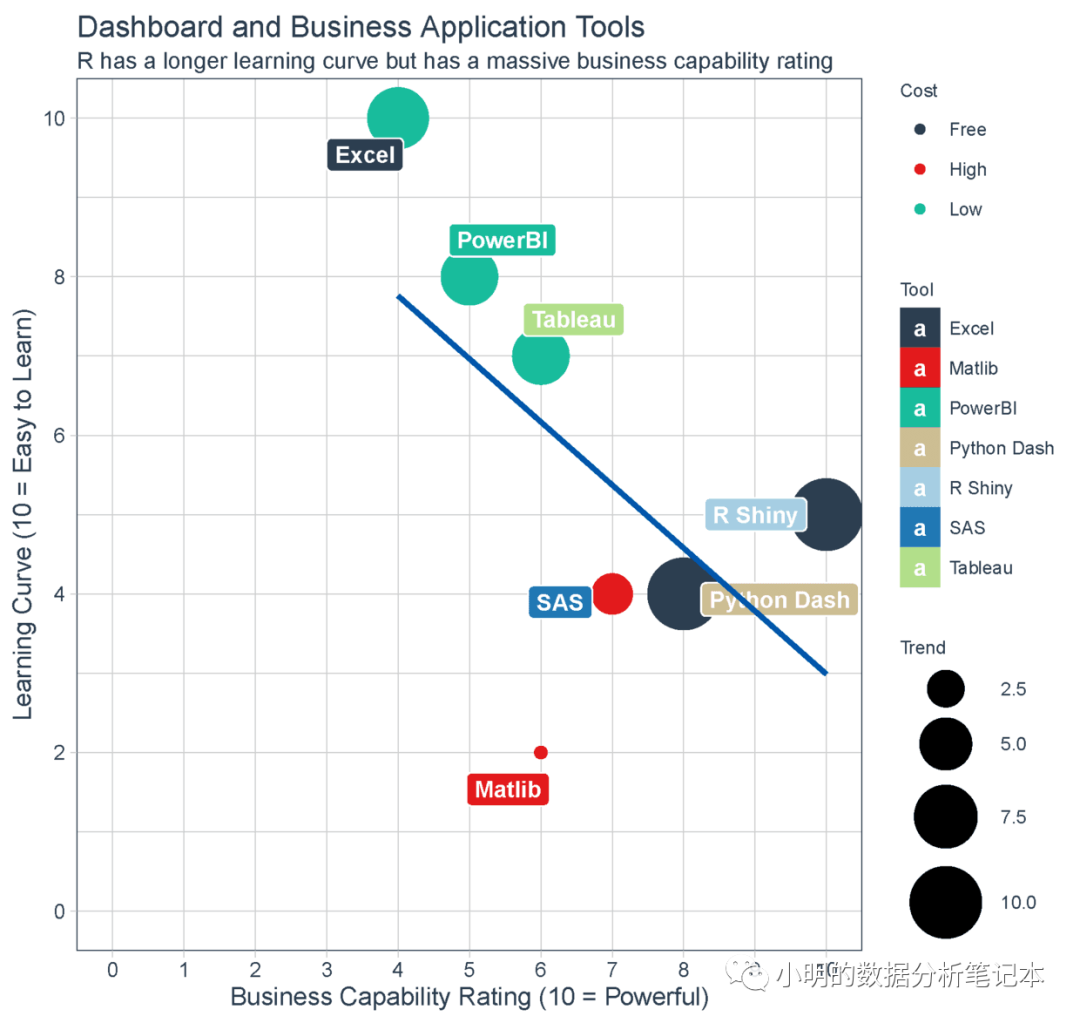
原文地址
https://www.r-bloggers.com/r-shiny-vs-tableau-3-business-application-examples/
作图代码
构造数据
data_apps<-data.frame(application=c("R Shiny","Python Dash","Excel","Tableau","PowerBI","Matlib","SAS"),
business_capability=c(10,8,4,6,5,6,7),
easy_of_learning=c(5,4,10,7,8,2,4),
trend=c(10,10,7,6,6,1,3),
cost=c("Free","Free","Low","Low","Low","High","High"))
加载需要的包
library(ggplot2)
library(ggrepel)
#install.packages("tidyquant")
library(tidyquant)
画图
ggplot(data_apps,aes(x=business_capability,y=easy_of_learning,
color=cost,size=trend))+
geom_point()+
geom_label_repel(aes(label=application,fill=application),
size=3.5,fontface="bold",color="white",
box.padding = 0.1,point.padding = 0.5,
segment.color = ''grey50'',segment.size = 1)+
geom_smooth(color=palette_dark()[[1]],method = "lm",se=F,show.legend = F)+
expand_limits(x=c(0,10),y=c(0,10))+
theme_tq()+
theme(legend.direction = "vertical",
legend.position = "right",
legend.title = element_text(size=8),
legend.text = element_text(size=8))+
scale_fill_tq()+
scale_color_tq()+
scale_y_continuous(breaks = seq(0, 10, 2)) +
scale_x_continuous(breaks = 0:10) +
scale_size_continuous(range = c(2, 14)) +
labs(title = "Dashboard and Business Application Tools",
subtitle = "R has a longer learning curve but has a massive business capability rating",
x = "Business Capability Rating (10 = Powerful)",
y = "Learning Curve (10 = Easy to Learn)",
color = "Cost",
size = "Trend",
fill = "Tool")
tidyquant
这个包时第一次使用,上面的代码中palette_dark()
、theme_tq()
、scale_fill_tq()
、scale_color_tq()
都是来自这个包中的函数。
使用help(package="tidyquant")
查看帮助文档 看到了一句话
Bringing financial and business analysis to the tidyverse
这个包应该是应用于金融领域的。
对于我来说可能是会用到这里的主题和配色方案!
接下来看一个帮助文档的例子https://business-science.github.io/tidyquant/reference/theme_tq.html
代码是
library(tidyquant)
library(dplyr)
library(ggplot2)
AAPL <- tq_get("AAPL", from = "2013-01-01", to = "2016-12-31")
AAPL
write.csv(AAPL,file="AAPL.csv",row.names = F,quote = F)
AAPL %>% ggplot(aes(x = date, y = close)) +
geom_line() +
geom_bbands(aes(high = high, low = low, close = close),
ma_fun = EMA,
wilder = TRUE,
ratio = NULL,
n = 50) +
coord_x_date(xlim = c("2016-01-01", "2016-12-31"),
ylim = c(75, 125)) +
labs(title = "Apple BBands",
x = "Date",
y = "Price") +
theme_tq()
重复这个例子获取数据可能需要科学上网,需要这个数据集的可以给我的公众号留言!
还用一个函数是palette_dark()
包括12个颜色 画个柱形图看一下
df<-data.frame(x=LETTERS[1:12],y=1:12)
x1<-palette_dark()
matrix(x1)[,1]
p1<-ggplot(df,aes(x,y))+
geom_col(aes(fill=x))+
theme_bw()+
scale_fill_manual(values = matrix(x1)[,1])+
ggtitle("palette_dark()")+
labs(x="",y="")+
theme(legend.position = "none")
x2<-palette_green()
p2<-ggplot(df,aes(x,y))+
geom_col(aes(fill=x))+
theme_bw()+
scale_fill_manual(values = matrix(x2)[,1])+
ggtitle("palette_green()")+
labs(x="",y="")+
theme(legend.position = "none")
x3<-palette_light()
p3<-ggplot(df,aes(x,y))+
geom_col(aes(fill=x))+
theme_bw()+
scale_fill_manual(values = matrix(x3)[,1])+
ggtitle("palette_light()")+
labs(x="",y="")+
theme(legend.position = "none")
cowplot::plot_grid(p1,p2,p3,ncol=1,align = "v")
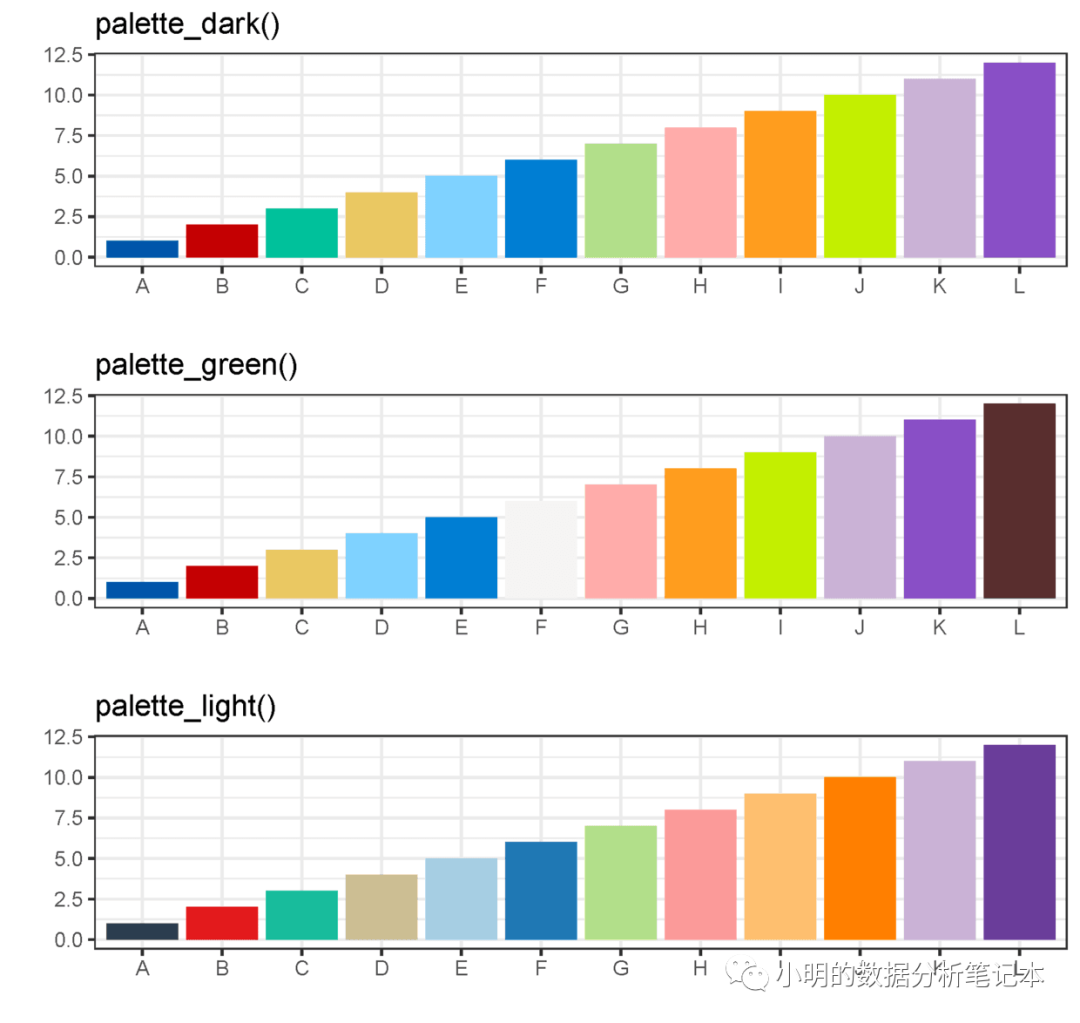
这个配色很好看!可以作为备选! 这三个函数产生的颜色好像是一样的,只是顺序不一样!
欢迎大家关注我的公众号
小明的数据分析笔记本
本文分享自微信公众号 - 小明的数据分析笔记本()。
如有侵权,请联系 support@oschina.cn 删除。
本文参与“OSC源创计划”,欢迎正在阅读的你也加入,一起分享。
ggplot,牛图;带有边际直方图的散点图:轴不匹配
如何解决ggplot,牛图;带有边际直方图的散点图:轴不匹配?
我正在寻找一种方法来创建带有边际直方图的散点图。 我找到了一个使用 ggplot2 + cowplot 的解决方案(感谢 @crsh):
library(ggplot2)
library(cowplot)
# Set up scatterplot
scatterplot <- ggplot(iris,aes(x = Sepal.Length,y = Sepal.Width,color = Species)) +
geom_point(size = 3,alpha = 0.6) +
guides(color = FALSE) +
theme(plot.margin = margin())
# Define marginal histogram
marginal_distribution <- function(data,var,group) {
ggplot(data,aes_string(x = var,fill = group)) +
geom_histogram(bins = 30,alpha = 0.4,position = "identity") +
# geom_density(alpha = 0.4,size = 0.1) +
guides(fill = FALSE) +
#theme_void() +
theme(plot.margin = margin())
}
# Set up marginal histograms
x_hist <- marginal_distribution(iris,"Sepal.Length","Species")
y_hist <- marginal_distribution(iris,"Sepal.Width","Species") +
coord_flip()
# Align histograms with scatterplot
aligned_x_hist <- align_plots(x_hist,scatterplot,align = "v")[[1]]
aligned_y_hist <- align_plots(y_hist,align = "h")[[1]]
# Arrange plots
plot_grid(
aligned_x_hist,NULL,aligned_y_hist,ncol = 2,nrow = 2,rel_heights = c(0.2,1),rel_widths = c(1,0.2)
)
我得到了:
现在,我有一些问题:
a) 如何在不破坏 plot_grid
的情况下添加图例和标题?
b) 散点图的轴与直方图的轴不匹配。我该如何解决这个问题?
问候!
解决方法
好的,我解决了轴问题:
我根据直方图的限制为散点图设置了 xlim
和 ylim
:
scatterplot <- scatterplot +
xlim(layer_scales(x_hist)$x$range$range) +
ylim(layer_scales(y_hist)$x$range$range)
但是我不知道如何添加标题和图例
Matplotlib 可视化50图:散点图(1)
导读
本系列将持续更新50个matplotlib
可视化示例,主要参考Selva Prabhakaran
在MachineLearning Plus
上发布的博文:Python可视化50图。
定义
关联图是查看两个事物之间关系的图像,它能够展示出一个事物随着另一个事物是如何变化的。关联图的类型有:折线图,散点图,相关矩阵等。
散点图
测试
- 导入需要使用的库
import numpy as np
import pandas as pd
import matplotlib as mpl
import matplotlib.pyplot as plt
import seaborn as sns
- plt.scatter
#绘制超简单的散点图:变量x1与x2的关系
#定义数据
x1 = np.random.randn(10) #取随机数
x2 = x1 + x1**2 - 10
#确定画布 - 当只有一个图的时候,不是必须存在
plt.figure(figsize=(8,4))
#绘图
plt.scatter(x1,x2 #横坐标,纵坐标
,s=50 #数据点的尺寸大小
,c="red" #数据点的颜色
,label = "Red Points"
)
#装饰图形
plt.legend() #显示图例
plt.show() #让图形显示
- 例子
# 除了两列X之外,还有标签y的存在
# 在机器学习中,经常使用标签y作为颜色来观察两种类别的分布的需求
X = np.random.randn(10,2) # 10行,2列的数据集
y = np.array([0,0,1,1,0,1,0,1,0,0])
colors = ["red","black"] # 确立颜色列表
labels = ["Zero","One"] # 确立标签的类别列表
for i in range(X.shape[1]):
plt.scatter(X[y==i,0],
X[y==i,1],
c=colors[i],
label = labels[i])
# 在标签中存在几种类别,就需要循环几次,一次画一个颜色的点
plt.legend()
plt.show()
实战
数据
# 导入数据
midwest = pd.read_csv("https://raw.githubusercontent.com/selva86/datasets/master/midwest_filter.csv")
# 探索数据
midwest.shape
midwest.head()
midwest.columns
标签
midwest[''category'']
# 提取标签中的类别
categories = np.unique(midwest[''category'']) # 去掉所有重复的项
categories # 查看使用的标签,如下图
颜色
plt.cm.tab10()
用于创建颜色的十号光谱,在 matplotlib
中,有众多光谱供我们选择:https://matplotlib.org/stable... 。可以在plt.cm.tab10()
中输入任意浮点数,来提取出一种颜色。光谱tab10中总共只有十种颜色,如果输入的浮点数比较接近,会返回类似的颜色。这种颜色会以元祖的形式返回,表示为四个浮点数组成的RGBA色彩空间或者三个浮点数组成的RGB色彩空间中的随机色彩。
color1 = plt.cm.tab10(5.2)
color1 # 四个浮点数组成的一个颜色
绘图
# 预设图像的各种属性
large = 22; med = 16; small = 12
params = {''axes.titlesize'': large, # 子图上的标题字体大小
''legend.fontsize'': med, # 图例的字体大小
''figure.figsize'': (16, 10), # 图像的画布大小
''axes.labelsize'': med, # 标签的字体大小
''xtick.labelsize'': med, # x轴上的标尺的字体大小
''ytick.labelsize'': med, # y轴上的标尺的字体大小
''figure.titlesize'': large} # 整个画布的标题字体大小
plt.rcParams.update(params) # 设定各种各样的默认属性
plt.style.use(''seaborn-whitegrid'') # 设定整体风格
sns.set_style("white") # 设定整体背景风格
# 准备标签列表和颜色列表
categories = np.unique(midwest[''category''])
colors = [plt.cm.tab10(i/float(len(categories)-1)) for i in range(len(categories))]
# 建立画布
plt.figure(figsize=(16, 10) # 绘图尺寸
, dpi=100 # 图像分辨率
, facecolor=''w'' # 图像的背景颜色,设置为白色,默认也是白色
, edgecolor=''k'' # 图像的边框颜色,设置为黑色,默认也是黑色
)
# 循环绘图
for i, category in enumerate(categories):
plt.scatter(''area'', ''poptotal'',
data=midwest.loc[midwest.category==category, :],
s=20, c=np.array(colors[i]).reshape(1,-1), label=str(category))
# 对图像进行装饰
# plt.gca() 获取当前的子图,如果当前没有任何子图的话,就创建一个新的子图
plt.gca().set(xlim=(0, 0.12), ylim=(0, 80000)) # 控制横纵坐标的范围
plt.xticks(fontsize=12) # 坐标轴上的标尺的字的大小
plt.yticks(fontsize=12)
plt.ylabel(''Population'',fontsize=22) # 坐标轴上的标题和字体大小
plt.xlabel(''Area'',fontsize=22)
plt.title("Scatterplot of Midwest Area vs Population", fontsize=22) # 整个图像的标题和字体的大小
plt.legend(fontsize=12) # 图例的字体大小
plt.show()
欢迎Star -> 学习目录 <- 点击跳转
国内链接 -> 学习目录 <- 点击跳转
本文由mdnice多平台发布
今天关于Pandas/Pyplot中的散点图:如何按类别绘制和pyplot 散点图的讲解已经结束,谢谢您的阅读,如果想了解更多关于ggplot2 PCA散点图绘制、ggplot2~一幅漂亮的散点图和新学到的配色方案、ggplot,牛图;带有边际直方图的散点图:轴不匹配、Matplotlib 可视化50图:散点图(1)的相关知识,请在本站搜索。
本文标签: