如果您对在pandas数据列中访问total_seconds和感兴趣,那么这篇文章一定是您不可错过的。我们将详细讲解在pandas数据列中访问total_seconds的各种细节,并对进行深入的分析,
如果您对在pandas数据列中访问total_seconds和感兴趣,那么这篇文章一定是您不可错过的。我们将详细讲解在pandas数据列中访问total_seconds的各种细节,并对进行深入的分析,此外还有关于40期《Pandas数据处理与分析》|pandas基础笔记2|pandas 基础|joyfulPandas、PANDACU Expands Reach into the African and Southeast Asian Second-Hand Clothing Markets、Pandas DataFrame.to_sql()函数是否需要后续的commit()?、pandas数据分析 | pandas.DataFrame数据修改、索引设置、数据组合的实用技巧。
本文目录一览:- 在pandas数据列中访问total_seconds()(pandas访问某一单元格)
- 40期《Pandas数据处理与分析》|pandas基础笔记2|pandas 基础|joyfulPandas
- PANDACU Expands Reach into the African and Southeast Asian Second-Hand Clothing Markets
- Pandas DataFrame.to_sql()函数是否需要后续的commit()?
- pandas数据分析 | pandas.DataFrame数据修改、索引设置、数据组合
在pandas数据列中访问total_seconds()(pandas访问某一单元格)
我想在pandas数据框中创建一个新列,该列是从数据框开始起经过的时间。我正在将日志文件导入具有数据时间信息的数据帧,但是无法访问其中的total_seconds()
功能s_df[''delta_t'']
。如果我访问列(s_df[''delta_t''].iloc[8].total_seconds()
)的各个元素,则可以使用,但是我想用total_seconds()创建一个新列,而我的尝试失败了。
s_df[''t''] = s_df.index # s_df[''t] is a column of datetimes_df[''delta_t''] = ( s_df[''t''] - s_df[''t''].iloc[0]) # time since start of data frames_df[''elapsed_seconds''] = # want column s_df[''delta_t''].total_seconds()
答案1
小编典典使用.dt访问器:
s_df[''elapsed_seconds''] = s_df[''delta_t''].dt.total_seconds()
例:
In [82]:df = pd.DataFrame({''date'': pd.date_range(dt.datetime(2010,1,1), dt.datetime(2010,2,1))})df[''delta''] = df[''date''] - df[''date''].iloc[0]dfOut[82]: date delta0 2010-01-01 0 days1 2010-01-02 1 days2 2010-01-03 2 days3 2010-01-04 3 days4 2010-01-05 4 days5 2010-01-06 5 days6 2010-01-07 6 days7 2010-01-08 7 days8 2010-01-09 8 days9 2010-01-10 9 days10 2010-01-11 10 days11 2010-01-12 11 days12 2010-01-13 12 days13 2010-01-14 13 days14 2010-01-15 14 days15 2010-01-16 15 days16 2010-01-17 16 days17 2010-01-18 17 days18 2010-01-19 18 days19 2010-01-20 19 days20 2010-01-21 20 days21 2010-01-22 21 days22 2010-01-23 22 days23 2010-01-24 23 days24 2010-01-25 24 days25 2010-01-26 25 days26 2010-01-27 26 days27 2010-01-28 27 days28 2010-01-29 28 days29 2010-01-30 29 days30 2010-01-31 30 days31 2010-02-01 31 daysIn [83]:df[''total_seconds''] = df[''delta''].dt.total_seconds()dfOut[83]: date delta total_seconds0 2010-01-01 0 days 01 2010-01-02 1 days 864002 2010-01-03 2 days 1728003 2010-01-04 3 days 2592004 2010-01-05 4 days 3456005 2010-01-06 5 days 4320006 2010-01-07 6 days 5184007 2010-01-08 7 days 6048008 2010-01-09 8 days 6912009 2010-01-10 9 days 77760010 2010-01-11 10 days 86400011 2010-01-12 11 days 95040012 2010-01-13 12 days 103680013 2010-01-14 13 days 112320014 2010-01-15 14 days 120960015 2010-01-16 15 days 129600016 2010-01-17 16 days 138240017 2010-01-18 17 days 146880018 2010-01-19 18 days 155520019 2010-01-20 19 days 164160020 2010-01-21 20 days 172800021 2010-01-22 21 days 181440022 2010-01-23 22 days 190080023 2010-01-24 23 days 198720024 2010-01-25 24 days 207360025 2010-01-26 25 days 216000026 2010-01-27 26 days 224640027 2010-01-28 27 days 233280028 2010-01-29 28 days 241920029 2010-01-30 29 days 250560030 2010-01-31 30 days 259200031 2010-02-01 31 days 2678400
40期《Pandas数据处理与分析》|pandas基础笔记2|pandas 基础|joyfulPandas
目录
- 一、文件的读取和写入
- 基本数据结构
- 1. Series
- 2. DataFrame
- 方法一(data,index,columns)
- 方法二(data(columns),index)
- 常用基本函数
- 1. 汇总函数
- 2. 特征统计函数
- 3.唯一值函数
- 4. 替换函数
- 数值替换 round,abs,clip
- 练习
- 5.排序函数
- 6.apply 方法
- 四、窗口对象
- 1. 滑窗对象
- 练习2
- 2. 扩张窗口
- 五、练习
- Ex2:指数加权窗口
import numpy as np
import pandas as pd
查看pandas 版本pd.__version__
一、文件的读取和写入
-
文件的读取
pd.read_csv()#csv
pd.read_table()#txt
pd.read_excel()#excel
常用的公共参数
header=None# 第一行不作为列名
index_col#表示把某一列或几列作为索引
usecols#读取列的集合
parse_dates#表示需要转化为时间的列
nrows#读取的数据的行数
Attention:
读取txt文件的时候,经常遇到分隔符非空格的情况,read_table有个分割参数 sep,用户可以自定义分割符号,进行txt数据的读取.
sep是正则参数,|需要转义成|
pd.read_table('../data/my_table_special_sep.txt', sep=' \|\|\|\| ', engine='python')
- 数据的写入
df.to_csv('...',index=False)
#当索引没有任何意义的时候可以在保存的时候去除
***** to_csv可以保存txt文件,可以自定义分隔符sep参数,一般设置成制表符'\t'
如果要将表格转换成markdown和latex可以使用to_markdown和to_latex,需要安装tabulate包。
基本数据结构
1. Series
Series
一般由四个部分组成,分别是序列的值data
、索引index
(name)、存储类型dtype
、序列的名字name
。
其中,索引也可以指定它的名字,默认为空。
s=pd.Series(data=[100,'a',{'dic1':5}],
index=pd.Index(['id1':20,'third'],name='my_idx'),
dtype='object',
name='my_name')
#获取属性
s.values
s.index //s.index.name#获取索引的名字
s[index_item]#取出单个索引的值 如s['third']
s.dtype
s.name
s.shape
2. DataFrame
DataFrame在Series的基础上增加了列索引
方法一(data,index,columns)
data=[[1,'a',1.2],[2,'b',2.2],[3,'c',3.2]]
df=pd.DataFrame(data=data,
index=['row_%d'%i for i in range(3)], ⭐
columns=['col_0','col_1','col_2'])
方法二(data(columns),index)
一般而言,更多的时候会采用从列索引名到数据的映射来构造数据框,同时再加上行索引:
df=pd.Dataframe(data={'col_0':[1,2,3]
'col_1':list('abc'),
'col_2':[1.2,2.2,3.2]},
index=['row_%d'%i for i in range(3)])
索引
df['col_0']
df[['col_0','col_1']]
df['col_0']['row_0']
常用属性
df.values
df.index
df.columns
df.dtypes
df.shape
df.T
常用基本函数
1. 汇总函数
引入数据
df=pd.read_csv('')
上述列名依次代表学校、年级、姓名、性别、身高、体重、是否为转系生、体测场次、测试时间、1000米成绩,本章只需使用其中的前七列。
取出数据前7列
df=df[df.columns[:7]]
取出数据前2行
df.head(2)
取出数据后3行
df.tail(3)
info()返回表的信息概况
df.info()
**describe表中数据数值列对应的主要统计量 **
df.describe()
更全面的数据汇总 使用pandas-profiling
2. 特征统计函数
sum, mean, median, var, std, max, min
引入df.demo数据
df_demo=df[['Height','Weight']]
df_demo.mean()
df_demo.max()
df_demo.quantile(0.75)
df_demo.count()
df_demo.idxmax() #获取pandas中series最大值对应的索引。
# idxmin是对应的函数
3.唯一值函数
对序列使用unique,nunique可以分别其唯一值组成的列表和唯一值的个数
df['School'].unique()#唯一值组成的列表
df['School'].nunique()#求某列有多少种不同的数
df['School'].value_counts()#得到唯一值及其对应的频数
观察多个列组合的唯一值 drop_duplicates()
关键参数:
keep:
'first’保留第一次出现的所在行,
‘last’保留最后一行所在行,
False:把所有重复组合所在的行删除
df_demo=df[['Gender','Transfer','Name']]
df_demo.drop_duplicates(['Gender','Transfer'],keep='last')
df['School'].drop_duplicates() # 在Series上也可以使用
duplicated和drop_duplicates的功能类似,但前者返回了是否为唯一值的布尔列表,其keep参数与后者一致。
drop_duplicates等价于把duplicated为True的对应行剔除。
4. 替换函数
替换操作是针对某一个列进行的
pandas
中的替换函数可以归纳为三类:映射替换、逻辑替换、数值替换。
映射替换
其中映射替换包含replace方法、第八章中的str.replace方法,第九章中的cat.codes方法
在replace中,可以通过字典构造,或者传入两个列表来进行替换
df['Gender'].replace({'Female':0,'Male':1}).head()
df['Gender'].replace(['Female','Male'],[0,1]).head()
replace特殊方向替换
method参数
ffill:用前面一个最近的未被替换的值进行替换
bfill:使用后面最近的未被替换的值进行替换
正则替换使用str.replace()
当前版本下对于string类型的正则替换还存在bug,因此如有此需求,请选择str.replace进行替换操作
逻辑替换
s=pd.Series([-1,1.2345,100,-50])
s.where(s<0)
s.where(s<0,100)
s.mask(s<0)#替换满足条件的值为Nan
s.mask(s<10,100)#替换满足条件的值为100
#构造一个bool序列类型,替换满足条件相应的数据
#传入的条件只需是与被调用的Series索引一致的布尔序列
s_condition=pd.Series([True,False,False,True],index=s.index)
s.mask(s_condition,-50)
数值替换 round,abs,clip
它们分别表示按照给定精度round四舍五入、abs取绝对值和clip截断
s=pd.Series([-1,1.2345,100,-50])
s.round(2)
s.clip(0,2)#前两个数分别表示上下截断边界
练习
clip 中,超过边界的只能截断为边界值,如果要把超出边界的替换为自定义的值,应当如何做?
s.clip(0,2).replace({2:100,0:-100})
5.排序函数
其一为值排序(sort_values),其二为索引排序(sort_index)
利用set_index
方法把年级和姓名两列作为索引
df_demo = df[['Grade', 'Name', 'Height', 'Weight']].set_index(['Grade','Name'])
df_demo.head(3)
#对身高进行排序,默认参数ascending=True为升序:
df_demo.sort_values('Height').head()
df_demo.sort_values('Height', ascending=False).head()
在排序中,经常遇到多列排序的问题,比如在体重相同的情况下,对身高进行排序,并且保持身高降序排列,体重升序排列:
df_demo.sort_values(['Weight','Height'],ascending=[True,False]).head()
索引排序的用法和值排序完全一致,只不过元素的值在索引中,此时需要指定索引层的名字或者层号,用参数level表示。
另外,需要注意的是字符串的排列顺序由字母顺序决定。
df_demo.sort_index(level=['Grade','Name'],ascending=[True,False]).head()
6.apply 方法
apply方法常用于DataFrame的行迭代或者列迭代
apply的参数往往是一个以序列为输入的函数
df_demo = df[['Height', 'Weight']]
def my_mean(x):
res = x.mean()
return res
df_demo.apply(my_mean)
=>
df_demo.apply(lambda x:x.mean())
=>
df_demo.apply(lambda x:x.mean(), axis=1).head()
mad函数返回的是一个序列中偏离该序列均值的绝对值大小的均值
df_demo.apply(lambda x:(x-x.mean()).abs().mean())
=>
df_demo.mad()
四、窗口对象
pandas中有3类窗口,分别是滑动窗口rolling、扩张窗口expanding以及指数加权窗口ewm
1. 滑窗对象
要使用滑窗函数,就必须先要对一个序列使用.rolling
得到滑窗对象,其最重要的参数为窗口大小window
。
roller使用的函数
s = pd.Series([1,2,3,4,5])
roller = s.rolling(window = 3)
roller. Mean()
roller.sum()
滑动相关系数或滑动协方差
roller.cov(s2)
roller.corr(s2)
支持使用apply传入自定义函数,其传入值是对应窗口的Series
roller.apply(lambda x:x.mean())
series使用的函数
shift, diff, pct_change是一组类滑窗函数,它们的公共参数为periods=n,默认为1,
分别表示取向前第n个元素的值、与向前第n个元素做差(与Numpy中不同,后者表示n阶差分)、
与向前第n个元素相比计算增长率。这里的n可以为负,表示反方向的类似操作。
s = pd.Series([1,3,6,10,15])
s.shift(2)
它们的功能可以用窗口大小为n+1的rolling方法等价代替:
s.rolling(3).apply(lambda x:list(x)[0]) # s.shift(2)
s.rolling(4).apply(lambda x:list(x)[-1]-list(x)[0]) # s.diff(3)
def my_pct(x):
L = list(x)
return L[-1]/L[0]-1
s.rolling(2).apply(my_pct) # s.pct_change()
练习2
rolling对象的默认窗口方向都是向前的,某些情况下用户需要向后的窗口,
例如对1,2,3设定向后窗口为2的sum操作,结果为3,5,NaN,此时应该如何实现向后的滑窗操作?
sd = pd.Series([1, 2, 3])
sd + sd[::-1].shift(1)
2. 扩张窗口
扩张窗口又称累计窗口,可以理解为一个动态长度的窗口,其窗口的大小就是从序列开始处到具体操作的对应位置,其使用的聚合函数会作用于这些逐步扩张的窗口上。具体地说,设序列为a1, a2, a3, a4,则其每个位置对应的窗口即[a1]、[a1, a2]、[a1, a2, a3]、[a1, a2, a3, a4]。
s = pd.Series([1, 3, 6, 10])
s.expanding().mean()
cummax, cumsum, cumprod函数是典型的类扩张窗口函数,请使用expanding对象依次实现它们
s.expanding().sum() # cummax()
s.expanding().max() # cumsum()
s.expanding().apply(lambda x:np.prod(x))# cumprod
五、练习
Ex1:口袋妖怪数据集
现有一份口袋妖怪的数据集,下面进行一些背景说明:
#代表全国图鉴编号,不同行存在相同数字则表示为该妖怪的不同状态
妖怪具有单属性和双属性两种,对于单属性的妖怪,Type 2为缺失值
Total, HP, Attack, Defense, Sp. Atk, Sp. Def, Speed分别代表种族值、体力、物攻、防御、特攻、特防、速度,其中种族值为后6项之和
-
对
HP, Attack, Defense, Sp. Atk, Sp. Def, Speed
进行加总,验证是否为Total
值。 -
对于
#
重复的妖怪只保留第一条记录,解决以下问题:
- 求第一属性的种类数量和前三多数量对应的种类
- 求第一属性和第二属性的组合种类
- 求尚未出现过的属性组合
- 按照下述要求,构造
Series
:
- 取出物攻,超过120的替换为
high
,不足50的替换为low
,否则设为mid
- 取出第一属性,分别用
replace
和apply
替换所有字母为大写 - 求每个妖怪六项能力的离差,即所有能力中偏离中位数最大的值,添加到
df
并从大到小排序
Ex2:指数加权窗口
- 作为扩张窗口的
ewm
窗口
在扩张窗口中,用户可以使用各类函数进行历史的累计指标统计,但这些内置的统计函数往往把窗口中的所有元素赋予了同样的权重。事实上,可以给出不同的权重来赋给窗口中的元素,指数加权窗口就是这样一种特殊的扩张窗口。
其中,最重要的参数是alpha
,它决定了默认情况下的窗口权重为
w
i
=
(
1
−
α
)
i
,
i
∈
{
0
,
1
,
.
.
.
,
t
}
w_i=(1−\alpha)^i,i\in\{0,1,...,t\}
wi=(1−α)i,i∈{0,1,...,t},其中
i
=
t
i=t
i=t表示当前元素,
i
=
0
i=0
i=0表示序列的第一个元素。
从权重公式可以看出,离开当前值越远则权重越小,若记原序列为 x x x,更新后的当前元素为 y t y_t yt,此时通过加权公式归一化后可知:
y t = ∑ i = 0 t w i x t − i ∑ i = 0 t w i = x t + ( 1 − α ) x t − 1 + ( 1 − α ) 2 x t − 2 + . . . + ( 1 − α ) t x 0 1 + ( 1 − α ) + ( 1 − α ) 2 + . . . + ( 1 − α ) t \begin{split}y_t &=\frac{\sum_{i=0}^{t} w_i x_{t-i}}{\sum_{i=0}^{t} w_i} \\&=\frac{x_t + (1 - \alpha)x_{t-1} + (1 - \alpha)^2 x_{t-2} + ...+ (1 - \alpha)^{t} x_{0}}{1 + (1 - \alpha) + (1 - \alpha)^2 + ...+ (1 - \alpha)^{t}}\\\end{split} yt=∑i=0twi∑i=0twixt−i=1+(1−α)+(1−α)2+...+(1−α)txt+(1−α)xt−1+(1−α)2xt−2+...+(1−α)tx0
对于Series
而言,可以用ewm
对象如下计算指数平滑后的序列:
np.random.seed(0)
s = pd.Series(np.random.randint(-1,2,30).cumsum())
s.head()
s.ewm(alpha=0.2).mean().head()
请用expanding
窗口实现。
- 作为滑动窗口的
ewm
窗口
从第1问中可以看到,ewm
作为一种扩张窗口的特例,只能从序列的第一个元素开始加权。现在希望给定一个限制窗口n
,只对包含自身的最近的n
个元素作为窗口进行滑动加权平滑。请根据滑窗函数,给出新的wi
与yt
的更新公式,并通过rolling
窗口实现这一功能。
教程来源:http://joyfulpandas.datawhale.club/Content/ch2.html
PANDACU Expands Reach into the African and Southeast Asian Second-Hand Clothing Markets
PANDACU, a renowned used clothes supplier based in China, has successfully ventured into the second-hand clothing markets in Africa and Southeast Asia. With its extensive experience and expertise in the industry, PANDACU aims to provide high-quality second-hand clothing options to meet the growing demand in these regions.
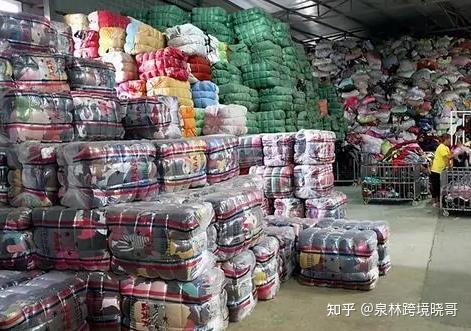
As a trusted name in the wholesale used clothes sector, PANDACU has built a solid reputation for its commitment to quality, reliability, and customer satisfaction. With its entry into the African and Southeast Asian markets, the company aims to cater to the diverse needs and preferences of retailers, resellers, and businesses operating in these regions.
The decision to expand into these markets comes as PANDACU recognizes the tremendous potential for growth and the increasing popularity of second-hand clothing among consumers. By offering a wide range of high-quality and affordable second-hand garments, PANDACU aims to tap into this demand and provide an extensive selection of clothing options for all genders and age groups.
PANDACU''s expansion into Africa and Southeast Asia is backed by its robust supply chain and logistics network, ensuring a seamless flow of products from its manufacturing facilities in China to the targeted markets. The company''s strong partnerships with reliable suppliers and manufacturers across China enable it to source a diverse range of clothing items, encompassing different styles, sizes, and seasons.
PANDACU''s foray into these markets is not only driven by its commitment to meeting customer demands but also by its desire to contribute to the sustainable fashion movement. By promoting the reuse and recycling of clothing, PANDACU aims to reduce the environmental impact of the fashion industry and foster a more sustainable and circular economy.
To ensure the utmost quality and customer satisfaction, PANDACU follows stringent quality control processes at every stage of its operations. From sorting and grading to cleaning and packaging, each garment undergoes thorough inspections to ensure it meets international quality standards. This meticulous approach guarantees that customers receive products that are durable, comfortable, and in excellent condition.
Moreover, PANDACU understands the importance of timely delivery and efficient service. The company''s dedicated team of professionals ensures smooth order processing, prompt shipment, and transparent communication throughout the entire transaction. By providing reliable and efficient service, PANDACU aims to establish long-term partnerships with customers in the African and Southeast Asian markets.
PANDACU''s expansion into Africa and Southeast Asia marks an important milestone in the company''s journey. By entering these markets, the company is poised to make a significant impact on the second-hand clothing industry, offering high-quality products and contributing to the sustainable fashion movement.
For further information or inquiries about PANDACU and its range of wholesale used clothes, please visit [website] or contact [contact information].
About PANDACU:
PANDACU is a leading used clothes supplier based in China, specializing in the wholesale distribution of high-quality second-hand clothing. With a commitment to quality, reliability, and sustainability, PANDACU serves retailers, resellers, and businesses worldwide, offering a diverse range of clothing options for all genders and age groups. Through its expansion into the African and Southeast Asian markets, PANDACU aims to meet the growing demand for second-hand clothing and promote a more sustainable fashion industry.
Pandas DataFrame.to_sql()函数是否需要后续的commit()?
to_sql()
适用于DataFrame
对象的Pandas函数的文档(请参阅to_sql()
文档)没有指出commit()
需要(或建议)对连接进行调用以保持更新。
我可以放心地假设这DataFrame.to_sql(''table_name'', con)
将始终自动提交更改(例如:)con.commit()
吗?
答案1
小编典典是的,一天结束时,它将自动提交。
熊猫调用SQLAlchemy方法executemany
(用于SQL Alchemy连接):
conn.executemany(self.insert_statement(), data_list)
对于SQLite连接:
def run_transaction(self): cur = self.con.cursor() try: yield cur self.con.commit() except: self.con.rollback() raise finally: cur.close()
并且由于SQL炼金术文档 executemany
的问题commit
末
pandas数据分析 | pandas.DataFrame数据修改、索引设置、数据组合
微信公众号:Python 集中营
简单的事情重复做,重复的事情坚持做,坚持的事情用心做;
你的肯定是我坚持的动力,如果这篇文章对你有帮助,点个关注吧!
相关扩展库
1# -*- coding: UTF-8 -*-
2
3import pandas as pd
4
5
6data_dict = {''first_col'': [1, 2, 3, 4], ''second_col'': [5, 6, 7, 8]}
7
8df = pd.DataFrame(data_dict)
数据修改
1import numpy as np
2
3# 转换某一列的数据类型
4
5df[''first_col'']=pd.DataFrame(df[''first_col''],dtype=np.float32)
6
7# 重新定义列名
8
9df.columns = [''first_col_1'',''second_col_1'']
10
11print(df)
12
13# 修改部分列名
14
15df.rename(columns = {''first_col_1'':''first_col_2'',''second_col_1'':''second_col_2''},inplace = True)
16
17print(df)
18
19# 按照某个或多个字段排序,ascending = False为降序、ascending = True为升序
20
21df = df.sort_values(by=[''first_col_2'',''second_col_2''],ascending = False)
22
23print(df)
24
25# 按照索引排序,ascending = False为降序、ascending = True为升序
26
27df = df.sort_index(axis = 0,ascending = True)
28
29print(df)
30
31# 按数据位置修改数据,如下将第二行、第二列数据修改为9
32
33df.iloc[1,1] = 9
34
35print(df)
36
37# 现有列计算生成新的列
38
39df[''third_col_2''] = df[''first_col_2''] + df[''second_col_2'']
40
41# first_col_2 second_col_2 third_col_2
42# 0 1.0 5 6.0
43# 1 2.0 9 11.0
44# 2 3.0 7 10.0
45# 3 4.0 8 12.0
索引设置
1# 重新设置索引
2
3df[''index'']=range(len(df[''first_col'']))
4
5df.set_index(df[''index''])
6
7print(df)
8
9# 设置日期为索引,定义日期范围:start开始日期、periods数据行数
10
11date = pd.date_range(start=''1/1/2021'',periods=len(df[''first_col'']))
12
13df = df.set_index(date)
14
15print(df)
数据连接与组合
1# 定义两个DataFrame数据
2
3df1 = pd.DataFrame(data_dict)
4
5df2 = pd.DataFrame(data_dict)
6
7# concat() 函数连接,axis=0表示当两个数据对象连接时存在不同的列不会生成新的列,axis=1表示会生成新的列
8
9df3=pd.concat([df1,df2],axis=0)
10
11print(df3)
12
13# 扩展函数append() 向df1中添加df2中的所有行、最后赋值给df3
14
15df3 = df1.append(df2.loc[:])
16
17print(df3)
DataFrame输出
1# excel保存
2
3df.to_excel(''/usr/data.xls'')
4
5# csv 保存
6
7df.to_csv(''/usr/data.csv'')
8
9# 输出字典形式
10
11dict_ = df.to_dict(orient="dict")
12
13print(dict_)
更多精彩前往微信公众号【Python 集中营】,专注于 python 技术栈,资料获取、交流社区、干货分享,期待你的加入~
关于在pandas数据列中访问total_seconds和的问题就给大家分享到这里,感谢你花时间阅读本站内容,更多关于40期《Pandas数据处理与分析》|pandas基础笔记2|pandas 基础|joyfulPandas、PANDACU Expands Reach into the African and Southeast Asian Second-Hand Clothing Markets、Pandas DataFrame.to_sql()函数是否需要后续的commit()?、pandas数据分析 | pandas.DataFrame数据修改、索引设置、数据组合等相关知识的信息别忘了在本站进行查找喔。
本文标签: